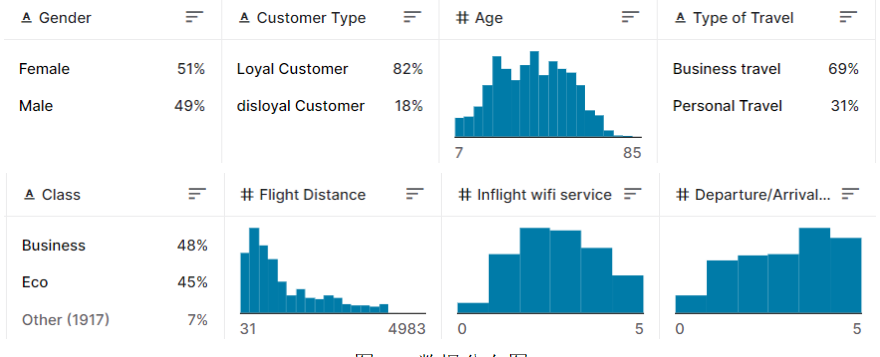
机器学习项目-基于随机森林的航空公司用户满意度分析
我们使用的数据来自 Kaggle 公开数据集,包含了航空公司乘客的基本信息、机票信息和满意度评分等,该数据中的乘客的部分特征如下:该数据集中共有约13万条数据,共包含25个变量,我们随机选择其中80%作为训练集,余下20%作为测试集。
·
摘要
航空旅行是人们出行的常用方式之一,乘客对于航空公司的服务质量有着较高的要求。满意度是衡量服务质量的重要指标,因此预测航空公司乘客的满意度对于提高服务质量具有重要意义。
近年来,机器学习在预测领域得到了广泛应用。机器学习模型具有自动学习能力,可以从数据中自动提取特征并进行预测,因此在预测航空公司乘客满意度方面具有较高的潜力。
本研究旨在通过使用机器学习模型来预测航空公司乘客的满意度。我们使用了 Kaggle 公开数据集,并对数据进行了缺失值补齐和特征放缩处理。在此基础上,我们使用了逻辑回归分类、支持向量机和随机森林分类器进行建模并调参。最后,我们对不同模型的性能进行了对比,并得出了有关航空公司乘客满意度的有用信息。
关键词:数据规范化,svm,随机森林,逻辑回归,决策树
数据集介绍
我们使用的数据来自 Kaggle 公开数据集,包含了航空公司乘客的基本信息、机票信息和满意度评分等,该数据中的乘客的部分特征如下:
该数据集中共有约13万条数据,共包含25个变量,我们随机选择其中80%作为训练集,余下20%作为测试集。
导入程序必要的库
pythoinimport pandas as pd
from sklearn import metrics
from sklearn.metrics import hinge_loss
from sklearn import svm
from sklearn.ensemble import RandomForestClassifier
from sklearn.preprocessing import StandardScaler
from sklearn.tree import DecisionTreeClassifier
读取训练集和测试集
X_train = pd.read_csv('dataset/train.csv')
X_test = pd.read_csv('dataset/test.csv')
X_train, X_test
数据预处理
def Preprocessing(X):
# 创建 StandardScaler 对象
scaler = StandardScaler()
# 3.1 将分类变量(非数值)转换为数值变量
X['Gender'] = X['Gender'].astype('category')
X['Gender'] = X['Gender'].astype('category').cat.codes # male:1 female:0
X['Customer Type'] = X['Customer Type'].astype('category')
X['Customer Type'] = X['Customer Type'].astype('category').cat.codes # disloyal Customer:1 Loyal Customer:0
X['Type of Travel'] = X['Type of Travel'].astype('category')
X['Type of Travel'] = X['Type of Travel'].astype('category').cat.codes # Personal Travel:1 Business travel:0
X['Class'] = X['Class'].astype('category')
X['Class'] = X['Class'].astype('category').cat.codes # Eco Plus:2 Business:1 Eco:0
X['satisfaction'] = X['satisfaction'].astype('category')
X['satisfaction'] = X['satisfaction'].astype('category').cat.codes # satisfied:1 neutral or dissatisfied:0
# 3.2 标准化
X_scaled = scaler.fit_transform(X[['Age']])
X['Age'] = X_scaled
X_scaled = scaler.fit_transform(X[['Flight Distance']])
X['Flight Distance'] = X_scaled
X_scaled = scaler.fit_transform(X[['Departure Delay in Minutes']])
X['Departure Delay in Minutes'] = X_scaled
X_scaled = scaler.fit_transform(X[['Arrival Delay in Minutes']])
X['Arrival Delay in Minutes'] = X_scaled
X = X.fillna(X.mean())
# 3.3 划分出训练数据 和 对应的label
return X.iloc[:,2:-1], X.iloc[:,-1]
测评指标
def evaluate(y_true, y_pred):
# 计算准确率
accuracy = metrics.accuracy_score(y_true, y_pred)
print("Accuracy:", accuracy)
# 计算精确率
precision = metrics.precision_score(y_true, y_pred)
print("Precision:", precision)
# 计算召回率
recall = metrics.recall_score(y_true, y_pred)
print("Recall:", recall)
# 计算 F1 值
f1 = metrics.f1_score(y_true, y_pred)
print("F1 score:", f1)
# 计算损失值
loss = hinge_loss(y_true, y_pred)
print("loss:", loss)
return accuracy, precision, recall, f1, loss
训练模型
数据处理、得到训练集和测试集
# 5.1数据处理、得到训练集和测试集
X_train, y_train = Preprocessing(X_train)
X_test, y_test = Preprocessing(X_test)
决策树模型
# 6.1 决策树模型
# 6.1.1初始化决策树分类器
clf = DecisionTreeClassifier()
# 6.1.2训练模型
clf.fit(X_train, y_train)
# 6.1.3使用模型进行预测
predictions = clf.predict(X_test)
# 6.1.4测评结果
evaluate(y_test, predictions)
随机森林分类器
# 6.2随机森林分类器
# 6.2.1创建随机森林分类器
clf = RandomForestClassifier(n_estimators=80, random_state=0)
# 6.2.2训练模型
clf.fit(X_train, y_train)
# 6.2.3预测结果
train_predictions = clf.predict(X_test)
#6.2.4测评模型
evaluate(y_test, train_predictions)
SVM
#6.3SVM 模型
# 6.3.1创建 SVM 模型, 样本均衡化
model = svm.SVC(kernel='linear', C = 1, class_weight='balanced')
# 6.3.2训练模型
model.fit(X_train, y_train)
# 6.3.3预测目标变量
predictions = model.predict(X_test)
https://mbd.pub/o/bread/ZpebmpZu
更多推荐
所有评论(0)