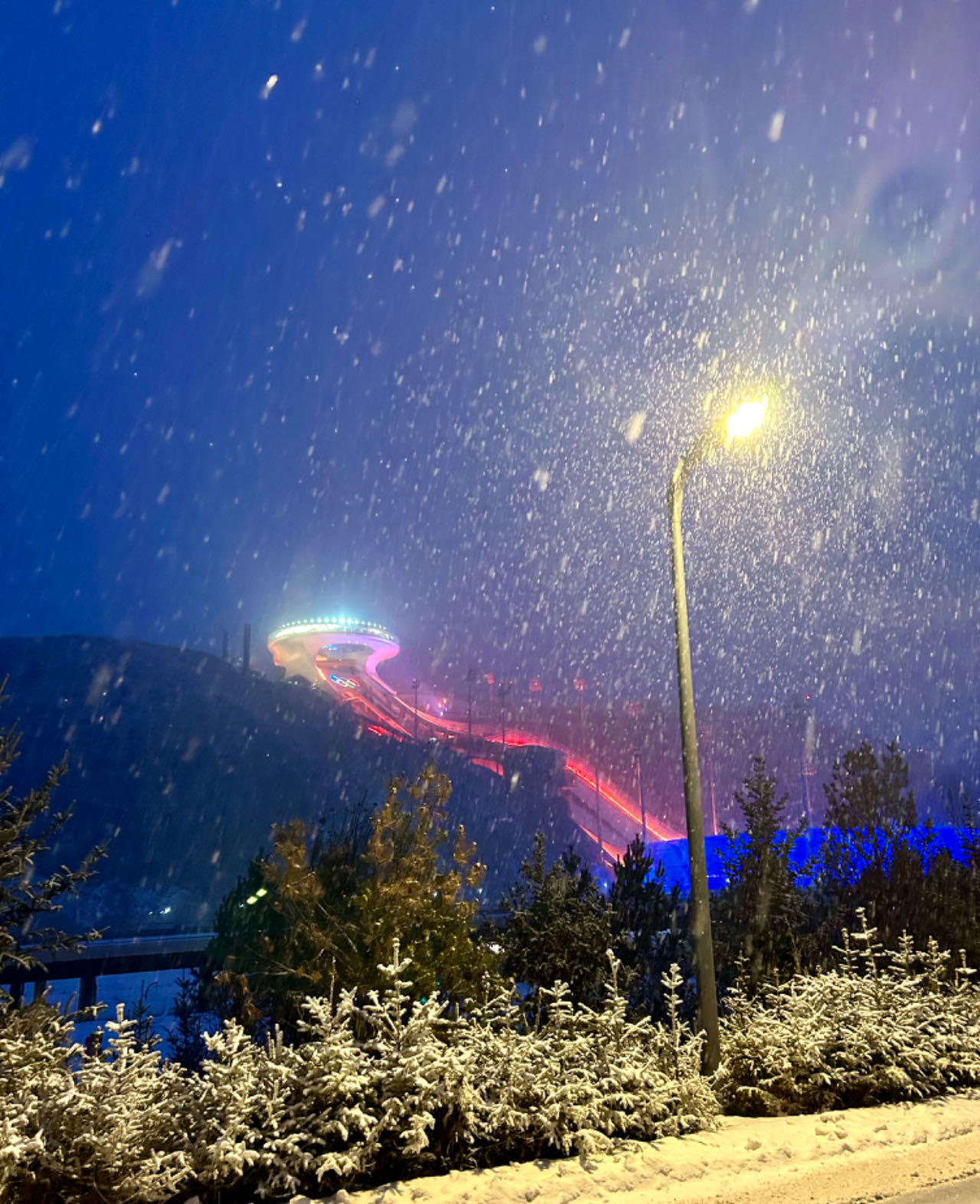
基于lora技术对Gemma(2B)大模型的微调实践
本文主要基于Lora技术,在Google colab上用A100对Gemma 2B大模型进行了指令微调,第一次指令微调是采用databricks-dolly-15k 作为数据集,取得了不错的微调效果,能准确用英文回答问题,但是databricks-dolly-15k 毕竟是英文数据集,微调后的模型对中文的理解并不好,为使模型对中文有更好的理解,笔者采用COIG-CQIA数据集进行了指令微调,微调
一、概述
本文主要基于Lora技术,在Google colab上用A100对Gemma 2B大模型进行了指令微调,第一次指令微调是采用databricks-dolly-15k 作为数据集,取得了不错的微调效果,能准确用英文回答问题,但databricks-dolly-15k 毕竟是英文数据集,微调后的模型对中文的理解并不好。为了使模型对中文有更好的理解,笔者采用COIG-CQIA数据集对模型进行了指令微调,并展示了微调前后的效果对比。
《两个数据集说明》
databricks-dolly-15k 是一个开源数据集,其中包含数千名 Databricks 员工在 InstructGPT 论文中概述的几个行为类别中生成的指令跟踪记录,包括头脑风暴、分类、封闭式 QA、生成、信息提取、开放式 QA 和摘要。
图:databricks-dolly-15k Dataset card
图:databricks-dolly-15k 具体内容
COIG-CQIA全称为Chinese Open Instruction Generalist - Quality is All You Need, 是一个开源的高质量指令微调数据集,旨在为中文NLP社区提供高质量且符合人类交互行为的指令微调数据。COIG-CQIA以中文互联网获取到的问答及文章作为原始数据,经过深度清洗、重构及人工审核构建而成。
图:COIG-CQIA-full Dataset card
图:COIG-CQIA-full 具体内容
二、前置条件
获得模型访问权,选择Colab运行时,配置训练环境。
先在Kaggle上注册,然后获得Gemma 2B 的访问权;
然后在Google colab 配置环境,主要是GPU的选择,免费的是T4,建议采用付费的A100(为了节省时间,微调训练耗时T4需要30分钟左右,A100只需要2分钟左右)
最后 在Kaggle 上的account上生成令牌文件(主要是usename 和 API Key),并将令牌文件配置到colab环境。
三、微调步骤
因为直接使用databricks-dolly-15k进行微调时,可以基于原有代码进行快速验证,为了采用COIG-CQIA-full数据集进行微调,最直接的想法就是把代码中databricks-dolly-15k部分替换为COIG-CQIA-full,然后对代码进行稍微修改,但这一步花费了笔者非常多的时间,因为无论如何修改,总会报错。最总采用了以下办法:
1、先通过代码上传COIG-CQIA-full代码,
2、然后将其转换为和databricks-dolly-15k同格式的内容,并将新内容命名为databricks-dolly-15k-fb,
3、然后下载到本地检测下内容是否正确。在正确的前提下,取databricks-dolly-15k-fb内容的前1600行,保存到另外一个文件databricks-dolly-15k-fb1,
4、最后使用databricks-dolly-15k-fb1进行微调。
通过此方法,可以基本不修改原有微调语料处理代码的前提下,完成微调训练。
图:COIG-CQIA-full 格式转化后内容
本次选择1600行,主要是为了减少上传的时间,当然也可以更少,openai建议的50行
Example count recommendations计数建议示例
To fine-tune a model, you are required to provide at least 10 examples. We typically see clear improvements from fine-tuning on 50 to 100 training examples with gpt-3.5-turbo but the right number varies greatly based on the exact use case.
要微调模型,您需要提供至少 10 个示例。我们通常会看到对 50 到 100 个训练示例进行微调的明显改进, gpt-3.5-turbo 但正确的数量会根据确切的用例而有很大差异。We recommend starting with 50 well-crafted demonstrations and seeing if the model shows signs of improvement after fine-tuning. In some cases that may be sufficient, but even if the model is not yet production quality, clear improvements are a good sign that providing more data will continue to improve the model. No improvement suggests that you may need to rethink how to set up the task for the model or restructure the data before scaling beyond a limited example set.
我们建议从 50 个精心制作的演示开始,看看模型在微调后是否显示出改进的迹象。在某些情况下,这可能就足够了,但即使模型尚未达到生产质量,明显的改进也是一个好兆头,表明提供更多数据将继续改进模型。没有改进表明,在扩展到有限的示例集之前,您可能需要重新考虑如何为模型设置任务或重组数据。
此外数据格式的检测也非常关键,openai官网有专门的格式检查代码。
Once you have compiled a dataset and before you create a fine-tuning job, it is important to check the data formatting. To do this, we created a simple Python script which you can use to find potential errors, review token counts, and estimate the cost of a fine-tuning job.
编译数据集后,在创建微调作业之前,检查数据格式非常重要。为此,我们创建了一个简单的 Python 脚本,您可以使用它来查找潜在错误、查看令牌计数以及估计微调作业的成本。
四、微调前后效果展示
基于databricks-dolly-15k微调的效果不做展示,主要展示下基于COIG-CQIA-full微调后的效果展示。
4.1微调前的表现
图:微调前问答表现
图:微调后问答表现
再看几个微调后的表现
图:微调后问答表现2
4.2微调前后训练参数对比
采用Lora微调,训练参数量由25亿降低到130万左右;
图:训练参数对比
4.3用A100微调训练的关键片段
训练耗时104s,80ms每步,这里如果采用T4,需要训练接近30分钟。
图:采用A100进行微调
五、关键源码
一、格式转换代码
import json
from google.colab import files # 如果你是在Google Colab环境中运行,需要导入该模块进行文件上传
# 提示上传文件
print("请上传 COIG-CQIA-full.jsonl 文件")
uploaded = files.upload()
# 获取上传文件名
uploaded_filename = list(uploaded.keys())[0]
# 读取上传的COIG-CQIA-full.jsonl文件
with open(uploaded_filename, "r", encoding="utf-8") as f:
coig_data = f.readlines()
# 转换格式
converted_data = []
for line in coig_data:
coig_entry = json.loads(line.strip())
converted_entry = {
"instruction": coig_entry["instruction"],
"context": coig_entry["input"],
"response": coig_entry["output"],
"category": "open_qa" if coig_entry["task_type"]["minor"] == ["问答"] else "classification"
}
converted_data.append(converted_entry)
# 将转换后的数据写入databricks-dolly-15k-fb.jsonl文件
with open("databricks-dolly-15k-fb.jsonl", "w", encoding="utf-8") as f:
for entry in converted_data:
f.write(json.dumps(entry, ensure_ascii=False) + "\n")
print("转换完成,结果已保存到 databricks-dolly-15k-fb.jsonl 文件中")
二、数据处理代码
import json
data = []
with open("databricks-dolly-15k-fb1.jsonl") as file:
for line in file:
features = json.loads(line)
# Filter out examples with context, to keep it simple.
if features["context"]:
continue
# Format the entire example as a single string.
template = "Instruction:\n{instruction}\n\nResponse:\n{response}"
data.append(template.format(**features))
# Only use 1000 training examples, to keep it fast.
data = data[:1000]
三、Lora微调代码
# Enable LoRA for the model and set the LoRA rank to 4.
gemma_lm.backbone.enable_lora(rank=4)
gemma_lm.summary()
# Limit the input sequence length to 512 (to control memory usage).
gemma_lm.preprocessor.sequence_length = 512
# Use AdamW (a common optimizer for transformer models).
optimizer = keras.optimizers.AdamW(
learning_rate=5e-5,
weight_decay=0.01,
)
# Exclude layernorm and bias terms from decay.
optimizer.exclude_from_weight_decay(var_names=["bias", "scale"])
gemma_lm.compile(
loss=keras.losses.SparseCategoricalCrossentropy(from_logits=True),
optimizer=optimizer,
weighted_metrics=[keras.metrics.SparseCategoricalAccuracy()],
)
gemma_lm.fit(data, epochs=1, batch_size=1)
参考文档:
更多推荐
所有评论(0)