一文讲解大模型的 Embedding 模型该如何进行微调?
本文重点介绍了如何使用 Sentence Transformers 对开源的Embedding模型进行微调,并验证Embedding模型微调后的效果。Sentence Transformers 是一个宝库,它介绍了关于Embedding模型方方面面的内容,是了解、深入Embedding模型必不可少的工具。后续笔者将会介绍Embedding模型量化、俄罗斯套娃嵌入模型(Matryoshka Repr
本文将会介绍如何使用 Sentence Transformers 对开源的Embedding模型bge-base-zh-v1.5
进行微调,并验证 Embedding 模型微调后的效果。
在RAG框架或者语义相似度计算任务时,Embedding模型是我们常常会打交道的模型。
Sentence Transformers
是一个 Python 库,用于使用和训练各种应用的Embedding模型,例如检索增强生成 (RAG)、语义搜索、语义文本相似度、释义挖掘 (paraphrase mining) 等等。其 3.0 版本的更新是该工程自创建以来最大的一次,引入了一种新的训练方法。
本文将会以智源研究院(BAAI)开源的Embedding模型bge-base-zh-v1.5
作为基准模型,展示如何使用Sentence Transformers
进行评估,并对其进行微调,验证微调后的模型效果会有所提升。
评估指标Baseline
使用LlamaIndex框架对RAG流程中的各种Retrieve算法,包括Embedding模型召回,进行了评估,评估指标采用Hit Rate
和MRR
。本文将继续使用这篇文章中给出的数据集进行评估。
示例评估代码如下:
# -*- coding: utf-8 -*-
# @file: bge_base_zh_eval.py
import os
import json
import time
import torch
from pprint import pprint
from sentence_transformers import SentenceTransformer
from sentence_transformers.evaluation import InformationRetrievalEvaluator
from sentence_transformers.util import cos_sim
project_dir = os.path.dirname(os.path.abspath(__file__)).split('/src')[0]
# data process
# load dataset, get corpus, queries, relevant_docs
with open(os.path.join(project_dir, "data/doc_qa.json"), "r", encoding="utf-8") as f:
content = json.loads(f.read())
corpus = content['corpus']
queries = content['queries']
relevant_docs = content['relevant_docs']
# # Load a model
# 替换成自己的模型完整路径或使用huggingface modl id
model_name = "bge-base-zh-v1.5"
model_path = os.path.join(project_dir, f"models/{model_name}")
model = SentenceTransformer(model_path, device="cuda" if torch.cuda.is_available() else "cpu")
print("Model loaded")
s_time = time.time()
# # Evaluate the model
evaluator = InformationRetrievalEvaluator(
queries=queries,
corpus=corpus,
relevant_docs=relevant_docs,
name=f"{os.path.basename(model_path)}",
score_functions={"cosine": cos_sim}
)
# Evaluate the model
result = evaluator(model)
pprint(result)
print(f"Time cost: {time.time() - s_time:.2f}s")
我们在评估器中传入queries, corpus, relevant_docs字典,加载完模型后即可进行评估。
评估结果在下文中给出,作为baseline(基准)指标。
微调数据合成
在LlamaIndex
框架中,可方便地使用generate_qa_embedding_pairs方法,利用Prompt工程对文本生成相关问题并进行关联。
Embedding模型的微调数据合成脚本如下:
# -*- coding: utf-8 -*-
# @file: make_ft_corpus.py
import os
from llama_index.legacy.finetuning import (
generate_qa_embedding_pairs
)
from llama_index.llms.openai import OpenAI
from llama_index.core import SimpleDirectoryReader
from llama_index.core.node_parser import SentenceSplitter
from dotenv import load_dotenv
load_dotenv()
project_dir = os.path.dirname(os.path.abspath(__file__)).split('/src')[0]
TRAIN_FILES = [os.path.join(project_dir, "data/ft_train.txt")]
VAL_FILES = [os.path.join(project_dir, "data/ft_test.txt")]
TRAIN_CORPUS_FPATH = os.path.join(project_dir, "data/ft_train_corpus.json")
VAL_CORPUS_FPATH = os.path.join(project_dir, "data/ft_val_corpus.json")
def load_corpus(files, verbose=False):
if verbose:
print(f"Loading files {files}")
reader = SimpleDirectoryReader(input_files=files)
docs = reader.load_data()
if verbose:
print(f"Loaded {len(docs)} docs")
parser = SentenceSplitter(chunk_size=250, chunk_overlap=0)
nodes = parser.get_nodes_from_documents(docs, show_progress=verbose)
if verbose:
print(f"Parsed {len(nodes)} nodes")
return nodes
train_nodes = load_corpus(TRAIN_FILES, verbose=True)
val_nodes = load_corpus(VAL_FILES, verbose=True)
llm = OpenAI(model="gpt-3.5-turbo", api_key=os.getenv("OPENAI_API_KEY"))
qa_generate_prompt_tmpl = """\
Context information is below.
---------------------
{context_str}
---------------------
Given the context information and not prior knowledge.
generate only questions based on the below query.
You are a Professor. Your task is to setup \
{num_questions_per_chunk} questions for an upcoming \
quiz/examination in Chinese. The questions should be diverse in nature \
across the document in Chinese. The questions should not contain options, not start with Q1/ Q2. \
Restrict the questions to the context information provided.
"""
train_dataset = generate_qa_embedding_pairs(nodes=train_nodes, llm=llm, num_questions_per_chunk=1, qa_generate_prompt_tmpl=qa_generate_prompt_tmpl)
val_dataset = generate_qa_embedding_pairs(nodes=val_nodes, llm=llm, num_questions_per_chunk=1, qa_generate_prompt_tmpl=qa_generate_prompt_tmpl)
train_dataset.save_json(TRAIN_CORPUS_FPATH)
val_dataset.save_json(VAL_CORPUS_FPATH)
输出结果如下:
Output:
Loading files ['/Users/admin/PycharmProjects/embedding_model_exp/data/ft_train.txt']
Loaded 1 docs
Parsing nodes: 100%|██████████| 1/1 [00:00<00:00, 23.54it/s]
Parsing nodes: 0%| | 0/1 [00:00<?, ?it/s]Parsed 137 nodes
Loading files ['/Users/admin/PycharmProjects/embedding_model_exp/data/ft_test.txt']
Loaded 1 docs
Parsing nodes: 100%|██████████| 1/1 [00:00<00:00, 45.84it/s]
0%| | 0/137 [00:00<?, ?it/s]Parsed 111 nodes
100%|██████████| 137/137 [03:34<00:00, 1.57s/it]
100%|██████████| 111/111 [01:55<00:00, 1.04s/it]
这样,我们就能得到微调数据集了,保存为ft_train_corpus.json和ft_val_corpus.json。
Embedding模型微调
接下来,我们将会对bge-base-zh-v1.5
模型进行微调,微调的目的是让模型更适配我们自己的数据集,从而取得更好的召回效果。
使用 `sentence-transformers v3`
这里,我们使用的sentence-transformers模块的版本为V3.0.0。
利用该模块,我们不难实现Embedding模型微调,微调代码如下:
# -*- coding: utf-8 -*-
# @file: ft_sentence_transformers_trainer.py
import os
import json
import time
import torch
from datasets import Dataset
from sentence_transformers import SentenceTransformer
from sentence_transformers.evaluation import InformationRetrievalEvaluator
from sentence_transformers.util import cos_sim
from sentence_transformers.losses import MultipleNegativesRankingLoss
from sentence_transformers import SentenceTransformerTrainingArguments
from sentence_transformers.training_args import BatchSamplers
from sentence_transformers import SentenceTransformerTrainer
start_time = time.time()
project_dir = os.path.dirname(os.path.abspath(__file__)).split('/src')[0]
# load eval dataset
with open(os.path.join(project_dir, "data/ft_val_dataset.json"), "r", encoding="utf-8") as f:
eval_content = json.loads(f.read())
corpus, queries, relevant_docs = eval_content['corpus'], eval_content['queries'], eval_content['relevant_docs']
# load train dataset
with open(os.path.join(project_dir, "data/ft_train_dataset.json"), "r", encoding="utf-8") as f:
train_content = json.loads(f.read())
train_anchor, train_positive = [], []
for query_id, context_id in train_content['relevant_docs'].items():
train_anchor.append(train_content['queries'][query_id])
train_positive.append(train_content['corpus'][context_id[0]])
train_dataset = Dataset.from_dict({"positive": train_positive, "anchor": train_anchor})
print(train_dataset)
print(train_dataset[0:5])
# Load a model
model_name = 'bge-base-zh-v1.5'
# 替换成自己的模型完整路径或使用huggingface modl id
model_path = os.path.join(project_dir, f"models/{model_name}")
model = SentenceTransformer(model_path, device="cuda:0" if torch.cuda.is_available() else "cpu")
print("Model loaded")
# # Evaluate the model
evaluator = InformationRetrievalEvaluator(
queries=queries,
corpus=corpus,
relevant_docs=relevant_docs,
name=f"{model_name}",
score_functions={"cosine": cos_sim}
)
train_loss = MultipleNegativesRankingLoss(model)
# define training arguments
args = SentenceTransformerTrainingArguments(
output_dir=f"ft_{model_name}", # output directory and hugging face model ID
num_train_epochs=5, # number of epochs
per_device_train_batch_size=2, # train batch size
gradient_accumulation_steps=2, # for a global batch size of 512
per_device_eval_batch_size=4, # evaluation batch size
warmup_ratio=0.1, # warmup ratio
learning_rate=2e-5, # learning rate, 2e-5 is a good value
lr_scheduler_type="cosine", # use constant learning rate scheduler
optim="adamw_torch_fused", # use fused adamw optimizer
tf32=True, # use tf32 precision
bf16=True, # use bf16 precision
batch_sampler=BatchSamplers.NO_DUPLICATES,
eval_strategy="epoch", # evaluate after each epoch
save_strategy="epoch", # save after each epoch
logging_steps=10, # log every 10 steps
save_total_limit=3, # save only the last 3 models
load_best_model_at_end=True, # load the best model when training ends
metric_for_best_model=f"eval_{model_name}_cosine_ndcg@10", # Optimizing for the best ndcg@10 score
)
# train the model
trainer = SentenceTransformerTrainer(
model=model, # the model to train
args=args, # training arguments
train_dataset=train_dataset.select_columns(
["positive", "anchor"]
), # training dataset
loss=train_loss,
evaluator=evaluator
)
trainer.train()
trainer.save_model()
print(f"cost time: {time.time() - start_time:.2f}s")
笔者在1张NVIDIA A800-SXM4-80GB型号的GPU上进行训练,耗时约63.10秒。同时,我们会将微调后的Embedding模型保存在GPU上。
总结
本文重点介绍了如何使用 Sentence Transformers 对开源的Embedding模型bge-base-zh-v1.5
进行微调,并验证Embedding模型微调后的效果。
Sentence Transformers 是一个宝库,它介绍了关于Embedding模型方方面面的内容,是了解、深入Embedding模型必不可少的工具。后续笔者将会介绍Embedding模型量化、俄罗斯套娃嵌入模型(Matryoshka Representation Learning, MRL)等相关方面的内容。
参考文献
-
Training and Finetuning Embedding Models with Sentence Transformers v3: https://huggingface.co/blog/train-sentence-transformers
-
Fine-tune Embedding models for Retrieval Augmented Generation (RAG): https://www.philschmid.de/fine-tune-embedding-model-for-rag
-
俄罗斯套娃 (Matryoshka) 嵌入模型概述: https://huggingface.co/blog/zh/matryoshka
-
Finetune Embeddings: https://docs.llamaindex.ai/en/stable/examples/finetuning/embeddings/finetune_embedding/
如何学习大模型 AI ?
由于新岗位的生产效率,要优于被取代岗位的生产效率,所以实际上整个社会的生产效率是提升的。
但是具体到个人,只能说是:
“最先掌握AI的人,将会比较晚掌握AI的人有竞争优势”。
这句话,放在计算机、互联网、移动互联网的开局时期,都是一样的道理。
我在一线互联网企业工作十余年里,指导过不少同行后辈。帮助很多人得到了学习和成长。
我意识到有很多经验和知识值得分享给大家,也可以通过我们的能力和经验解答大家在人工智能学习中的很多困惑,所以在工作繁忙的情况下还是坚持各种整理和分享。但苦于知识传播途径有限,很多互联网行业朋友无法获得正确的资料得到学习提升,故此将并将重要的AI大模型资料包括AI大模型入门学习思维导图、精品AI大模型学习书籍手册、视频教程、实战学习等录播视频免费分享出来。
第一阶段(10天):初阶应用
该阶段让大家对大模型 AI有一个最前沿的认识,对大模型 AI 的理解超过 95% 的人,可以在相关讨论时发表高级、不跟风、又接地气的见解,别人只会和 AI 聊天,而你能调教 AI,并能用代码将大模型和业务衔接。
- 大模型 AI 能干什么?
- 大模型是怎样获得「智能」的?
- 用好 AI 的核心心法
- 大模型应用业务架构
- 大模型应用技术架构
- 代码示例:向 GPT-3.5 灌入新知识
- 提示工程的意义和核心思想
- Prompt 典型构成
- 指令调优方法论
- 思维链和思维树
- Prompt 攻击和防范
- …
第二阶段(30天):高阶应用
该阶段我们正式进入大模型 AI 进阶实战学习,学会构造私有知识库,扩展 AI 的能力。快速开发一个完整的基于 agent 对话机器人。掌握功能最强的大模型开发框架,抓住最新的技术进展,适合 Python 和 JavaScript 程序员。
- 为什么要做 RAG
- 搭建一个简单的 ChatPDF
- 检索的基础概念
- 什么是向量表示(Embeddings)
- 向量数据库与向量检索
- 基于向量检索的 RAG
- 搭建 RAG 系统的扩展知识
- 混合检索与 RAG-Fusion 简介
- 向量模型本地部署
- …
第三阶段(30天):模型训练
恭喜你,如果学到这里,你基本可以找到一份大模型 AI相关的工作,自己也能训练 GPT 了!通过微调,训练自己的垂直大模型,能独立训练开源多模态大模型,掌握更多技术方案。
到此为止,大概2个月的时间。你已经成为了一名“AI小子”。那么你还想往下探索吗?
- 为什么要做 RAG
- 什么是模型
- 什么是模型训练
- 求解器 & 损失函数简介
- 小实验2:手写一个简单的神经网络并训练它
- 什么是训练/预训练/微调/轻量化微调
- Transformer结构简介
- 轻量化微调
- 实验数据集的构建
- …
第四阶段(20天):商业闭环
对全球大模型从性能、吞吐量、成本等方面有一定的认知,可以在云端和本地等多种环境下部署大模型,找到适合自己的项目/创业方向,做一名被 AI 武装的产品经理。
- 硬件选型
- 带你了解全球大模型
- 使用国产大模型服务
- 搭建 OpenAI 代理
- 热身:基于阿里云 PAI 部署 Stable Diffusion
- 在本地计算机运行大模型
- 大模型的私有化部署
- 基于 vLLM 部署大模型
- 案例:如何优雅地在阿里云私有部署开源大模型
- 部署一套开源 LLM 项目
- 内容安全
- 互联网信息服务算法备案
- …
学习是一个过程,只要学习就会有挑战。天道酬勤,你越努力,就会成为越优秀的自己。
如果你能在15天内完成所有的任务,那你堪称天才。然而,如果你能完成 60-70% 的内容,你就已经开始具备成为一名大模型 AI 的正确特征了。
这份完整版的大模型 AI 学习资料已经上传CSDN,朋友们如果需要可以微信扫描下方CSDN官方认证二维码免费领取【保证100%免费
】
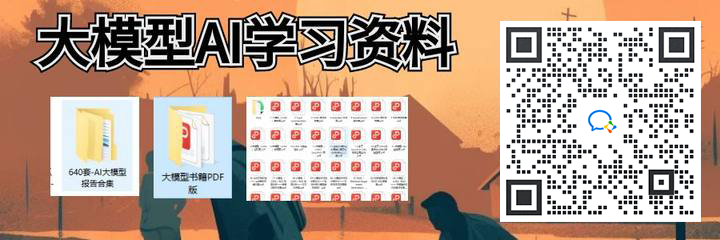
更多推荐
所有评论(0)