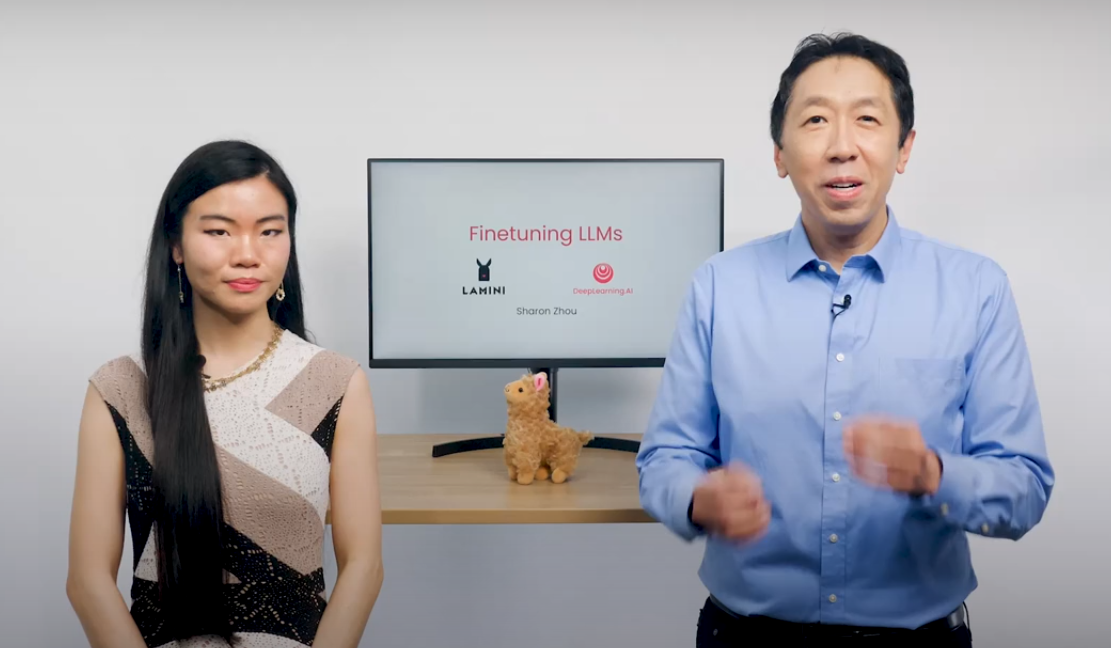
【LLM】吴恩达『微调大模型』课程完全笔记
『Finetuning Large Language Models』是DeepLearning.AI出品的免费课程,版权属于DeepLearning.AI(https://www.deeplearning.ai/)。本文是对该课程内容的翻译整理,只作为教育用途,不作为任何商业用途。
Finetuning Large Language Models
版权说明:
『Finetuning Large Language Models』是DeepLearning.AI出品的免费课程,版权属于DeepLearning.AI(https://www.deeplearning.ai/)。
本文是对该课程内容的翻译整理,只作为教育用途,不作为任何商业用途。
翻译整理:西安邮电大学 黄杉 (https://github.com/youcans)
吴恩达『微调大模型』课程完全笔记
## 0. 课程概述(Introduction)
通过编写prompt在多数场景下可以很好的让LLM遵循指令来执行任务,例如提取关键词、文本分类等。但是通过对LLM进行微调,可以使LLM更加稳定的执行想要的操作。
通过编写prompt让LLM以某种特定的语气回复,例如更贴心、更礼貌、更简洁,或者在某种程度上遵循特定的表达方式,可能还具有一定的挑战性。微调实际上也是调整语气的一种很好的方法。
现在人们已经意识到,ChatGPT和其它LLM在回答各种主题的问题方面具有惊人的能力,但是个人和公司希望将同样的能力应用于他们自己的私有和专有数据,实现这一目标的方式之一是使用你的数据训练LLM。
训练基础LLM需要大量的数据,可能是数千亿甚至上万亿的数据量,以及大量的GPU计算资源。但是通过微调,你可以在现有LLM上进一步训练你自己的数据。
在本课程,你将了解到:
- 什么是微调,在哪些情况下微调可能对你的应用有帮助;
- 微调如何融入训练,它与提示工程或者检索增强生成有何不同,以及如何将这些技术与微调结合使用;
- 深入探讨微调的特殊变体,这种方式已经将GPT3打造成了ChatGPT,称为指令微调,它教会了LLM遵循指令;
- 逐步了解如何微调自己的LLM,准备数据、训练模型,并在代码中进行评估。
本课程适合熟悉 Python 语言的同学。但如果要理解全部代码,还要了解一些深度学习的基本知识。例如,了解训练神经网络的过程,什么是训练/测试数据的分割。
很多人为这门课程付出了努力,他们是:Lamini 团队的 Nian Wei, DeepLearning.AI 团队的 Tommy Nelson, Geoff Ladwig。
通过这门简短的课程,你将深入了解如何微调现有的LLM,以及如何在你自己的数据上微调构建你的LLM。
1. 为什么要微调(Why finetune)
本节讨论什么是微调,将微调与提示工程进行比较,通过一个实验来比较微调模型与非微调模型。
1.1 什么是微调?
微调就是将这些通用大模型,例如:
- GPT3,专门用于像ChatGPT这样的特定用途的聊天机器人,使其在聊天中表现良好
- GPT4,并将其转变为专用的GitHub Copilot用例,以自动补全代码
- PCP,初级保健医生就像是你的通用模型,但是经过训练和微调的模型就像是心脏病专家或者皮肤科医生,一位拥有特定专业知识的医生,可以更深入地处理心脏问题或者皮肤问题
1.2 微调在做什么?
- 因此,微调对大模型的作用,是它使你能为LLM提供比prompt多的多的数据。
大模型可以从该数据中进行学习,而不仅仅是访问该数据,通过这个学习过程,它能够从PCP通用大模型升级为像皮肤科医生这样更专业的大模型。
如下图所示,你可能会遇到一些症状,将其输入模型,比如皮肤刺激、发红、瘙痒等。基础大模型可能会说这可能是粉刺,然而,如果在皮肤科数据上进行了微调的大模型接收相同的症状,就能为你提供更清晰、更具体的诊断。
- 除了学习新的信息之外,微调可以让大模型给出更加一致(consistent)的输出或行为。
例如,当提问通用大模型:what is your first name?它可能会回答:what is your last name?因为通用大模型看到过太多的问不同问题的调查问卷,所以它甚至不知道你是要它回答这个问题。但是一个经过微调的大模型,当你问它相同的问题时,它能够清晰地回复:My fisrt name is Sharon.
- 微调还可以帮助大模型减少幻觉(hallucinations)
这是一个常见的问题,大模型会胡乱编造一些东西。当这个模型没有基于我的数据训练,也许它会说我的名字是 Bob——我的名字肯定不是 Bob。
总之,微调使你能够将大模型定制到特定的用途。实际上,它与大模型早期的训练方法非常相似。
1.3 微调和提示工程的区别
下面我们来比较微调与提示工程。
提示工程(Prompt Engineering)
提示工程就像 Google搜索那样,只是输入一个查询并编辑查询来改变你所看到的结果。
提示工程有很多优点:
- 不需要任何数据就可以开始使用大模型,只需要开始聊天。
- 前期成本较低,每次与大模型通信都不会太昂贵,不需要考虑成本。
- 不需要技术知识就可以入门,只需要知道如何给大模型发送信息。
- 可以使用检索增强生成(RAG)或者BRAG,将更多的外部数据引入其中,并有选择的选取放入提示的数据类型。
提示工程也有很多缺点:
- 如果你拥有的数据不少,那么可能不适合放入提示中,所以不可能使用太多数据。
- 如果尝试装入大量数据,不幸的是,大模型将会忘记很多数据。
- 存在幻觉问题,也就是大模型会编造信息,而且很难纠正已经学到的错误信息。
- 虽然使用检索增强生成可以很好的引入自己的数据,但它也经常会错过正确的数据,获取错误的数据,并导致大模型输出错误的内容。
微调(Finetuning)
微调的优点是:
- 与提示工程相反,微调可以装入几乎无限量的数据
- 大模型可以从这些数据上学到新的信息。
- 因此可以纠正它之前可能学到的错误信息,甚至可以加入它之前没有了解过的最新信息。
- 如果对较小的模型进行微调,后期成本会比较低。如果以后会频繁使用模型,这一点很重要。
- 可以使用检索增强生成(RAG),可以将其与更多的数据连接起来,即使在学习了所有这些信息之后。
微调的缺点是:
- 需要更多高质量的数据。
- 前期计算成本较高,微调并不是免费的,也不是花几美元就可以开始的。当然,现在有一些免费工具可以使用,但这个过程是需要大量算力的。
- 需要具备一些技术知识,将数据放在正确的地方。虽然现在有越来越多的工具使这个过程更加容易,但仍然需要对那些数据有一些理解。
总结
- 提示工程的通用性很好,它非常适合应用于辅助功能和项目初期原型设计,非常适合快速入门。
- 微调非常适合应用于企业或特定领域的场景,是面向真实生产的应用。而且具有隐私性。
1.4 微调的优势
性能方面
- 可以减少幻觉,阻止LLM胡言乱语。
- 可以增加更多的领域专业知识。
- 可以增强输出的一致性和可靠性,减少输出不想要的信息,使其在适配性方面更好。
隐私方面
- 可以在VPC或本地训练。
- 可以防止在现成的第三方解决方案上可能发生的数据泄漏和数据违规。
- 是一种保护私有数据的方法。
成本方面
- 微调较小的LLM可以降低每次请求的成本。
- 可以增加成本的透明度。
- 在成本以及其它方面都有更大的控制权,例如正常运行时间(uptime)和延迟(latency)等。
可靠性方面
- 运行时间可控(uptime)
- 可以极大减少特定应用程序的延迟(lower latency),例如代码自动补全,延迟可能需要低于200ms
- 在适应性(moderation)方面,如果你希望大模型为某些事情道歉,或者对某些事情表示不知道,甚至拥有自定义的回应,微调可以向大模型提供这些限制。
1.5 实验 Lab1:比较微调模型与非微调模型
我们进行一个微调模型的实验。
模型微调需要 3 个Python 库的支持:
- Pytorch,这是最底层的接口;
- Huggingface,这是基于Pytorch做了更高层次的抽象,可以轻松导入数据集并训练数据;
- LLama Library(Lamini),这是更高级的接口,只需三行代码即可训练大模型。
下面是一个基于LLama-2-7B大模型的示例:Tell me how to train my dog to sit。我们将会比较一个 Fine-Tune 微调模型和一个Non-Fine-Tune 非微调模型。
首先,从llama库导入基本模型运行器,这个类帮助我们运行开源模型,可以高效地运行我们托管在 GPU 上的开源模型。
你可以运行的第一个模型是 llama tube 模型,这是一个非微调模型,只是基于HuggingFace名称来实例化。我们直接进行询问,并打印输出。输出结果说明,这个模型实际上并没有被训练成对那个命令进行回应。效果比较糟糕,没有对问题进行正确的回应。
然后,我们将其与经过微调以进行实际聊天的llama2进行比较。实例化经过微调的模型,然后对这个经过微调的模型同样的询问,并打印输出。我们立刻就能看出区别,效果好多了,逐步列出训练狗坐下的步骤。
使用 [INST] 标记可以告诉模型这部分内容是我的指令。
最后,我们调用 chatGPT,用同样的询问来进行测试。尝试用 chatGPT 或其它模型来看看它们的效果,进行比较。
总之,经过微调的模型,包括chatGPT 和 LaminiChatLLM,显然比没有经过微调的模型的性能好得多。
我们可以发现,经过微调的模型,不管是chatGPT还是llama,它们显然比那个没有经过微调的要好。
- 简明的例程:
#直接从llama库中导入
from llama import BasicModelRunner
finetuned_model=BasicModelRunner("meta-llama/llama-2-7b-chat-hf")
finetuned_output=finetuned_model("Tell me how to train my dog to seat")
#引入chatGPT的话
chatGPT=BasicModelRunner("chat-gpt")
- 本教程的完整例程:
from llama import BasicModelRunner
"""Try Non-Finetuned models"""
non_finetuned = BasicModelRunner("meta-llama/Llama-2-7b-hf")
non_finetuned_output = non_finetuned("Tell me how to train my dog to sit")
print(non_finetuned_output)
print(non_finetuned("What do you think of Mars?"))
print(non_finetuned("taylor swift's best friend"))
print(non_finetuned("""Agent: I'm here to help you with your Amazon deliver order.
Customer: I didn't get my item
Agent: I'm sorry to hear that. Which item was it?
Customer: the blanket
Agent:"""))
"""Compare to finetuned models"""
finetuned_model = BasicModelRunner("meta-llama/Llama-2-7b-chat-hf")
finetuned_output = finetuned_model("Tell me how to train my dog to sit")
print(finetuned_output)
print(finetuned_model("[INST]Tell me how to train my dog to sit[/INST]"))
print(non_finetuned("[INST]Tell me how to train my dog to sit[/INST]"))
print(finetuned_model("What do you think of Mars?"))
print(finetuned_model("taylor swift's best friend"))
print(finetuned_model("""Agent: I'm here to help you with your Amazon deliver order.
Customer: I didn't get my item
Agent: I'm sorry to hear that. Which item was it?
Customer: the blanket
Agent:"""))
2. 微调适用于哪些问题(Where finetuning fits in)
本节中我们讨论如何在训练过程中进行微调,微调可以应用于哪些不同的任务。
2.1 预训练
预训练是微调发生之前的第一步。
- 在预训练之前,模型是完全随机的。
模型对世界一无所知,因此模型的所有权重都是完全随机的。模型还没有语言能力,无法生成英文单词。
模型的学习目标,是预测下一个token。简单的说,这只是预测下一个单词(word)。但开始的时候模型没有语言能力,无法正确输出。
大模型训练过程,是从大量的文本语料数据中获取知识。
通常是从整个网络中获取的,我们称之为“无标签数据(unlabeled data)”,因为并不是组织好的,而是从网络上获取的。
要让数据集能有效地用于模型预训练,仍然需要大量的人工工作。这通常被称为自监督学习(self-supervised learning),因为模型本质上是通过预测下一个token在进行自监督学习。它要做的就是预测下一个词,实际上并没有任何标签。
- 在大模型预训练之后
经过训练,模型学会了语言,能够预测下一个词语或 token。模型从互联网中学会了大量的知识。
这样的方法能够有效,是难以置信的。模型所做的一切,就是尝试预测下一个 token,并通过阅读整个互联网的数据来完成这个任务。
大模型从互联网上抓取了什么数据,背后的数据和知识往往是不公开的。我们并不知道那些大公司的闭源模型使用的数据集是什么。
LutherAI创建了一个名为"The Pile"的数据集,涵盖了整个互联网上抓取的22个不同的数据集。例如,既有林肯的演说,也有蛋糕的食谱,有从PubMed上获取的关于医学文本的信息,还有来自 GitHub的代码。通过将它们组织在一起,为模型注入了知识。
这个预训练过程是非常昂贵且耗时,因为它要让模型在所有数据中进行训练,它要从绝对的随机性逐渐理解这些文本。所以这些预训练基础模型非常棒。
2.2 预训练的局限性
实际上有很多这样的开源模型。它是从网络上获取的数据集上进行训练的。
训练数据可能如下图左侧所示。受到训练数据的影响,它并不一定适合作为一个聊天机器人。例如,如果问大模型"墨西哥的首都是哪里?“,大模型可能会输出"匈牙利的首都是哪里?”,从一个聊天机器人的角度来看,这实际上并没有多大用处。
2.3 预训练之后的微调
预训练是让你得到基础模型的第一步。如何将其打造成为一个聊天机器人呢?将通用大模型打造成一个聊天机器人,微调是一个重要的方法。
当你需要添加数据时,可以使用微调来获得一个经过微调的模型。对于一个经过微调的模型,还可以继续添加微调步骤。
微调通常涉及到更深入的训练。可以从不同来源获取未标记数据,并将其整合在一起进行自监督学习。也可以是自己整理的带标签的数据,使其更加结构化,以便大模型学习。
微调和预训练的不同之处,就是微调需要的数据要少得多。你是在这个已经学习了很多知识和基本语言技能的基础模型上进行微调,你只是将它带到了一个新的水平,并不需要那么多的数据。
如果你来自机器学习的其它任务,例如你在处理图像,并且已经在 ImageNet 上进行了微调。你会发现这里对于大模型的微调的定义更加宽松。实际上是在更新整个模型的权重,而不仅仅是一部分。
微调的目的和预训练是一样的:预测下一个token。我们所做的只是改变数据,使其以一种更结构化的方式呈现,使大模型可以在输出和模仿某种数据结构时更加一致(consistent)
还有更好的方法,允许以最小的代价来更新你的模型,我们稍后再进行讨论。
2.4 微调在做什么
- 改变行为
-
具有更好的一致性
你正在改变模型的行为。你在确切地告诉它,我们正处在聊天界面中,而不是在看调查问卷。这会使模型的回答具有更好的一致性(consistently)。 -
具有更好的适应性
大模型可以更好的集中注意力,例如适应性(moderation)。
-
这通常只是在挖掘大模型的能力,例如更好的对话能力。对比在微调之前,为了从大模型中获取需要的信息,我们不得不进行大量的提示工程。
-
获取知识
- 可以增加基础模型在预训练过程中没有的新的特定主题的知识
- 可以纠正旧的不正确的信息,使模型融入最新的信息
-
改变行为并获取知识
通常情况下,这是更加常见的。改变其行为,并获取新的知识。
2.5 要进行微调的任务有哪些
文本输入、文本输出:对于LLM来说,实际上只是输入文本、输出文本。我们将任务分为两类,一类是提取文本,另一类是扩展文本。
-
提取文本/摘要(Extraction)
- 阅读
- 提取关键词、主题
-
扩展(Expansion)
- 写作
- 聊天、写邮件、写代码
模型能明确理解这两个任务之间的区别,或者多个需要微调的任务的区别,是能够成功的关键。如果你想要在微调任务上取得成功,就要明确你想要做什么任务。
“清晰(Clarity)”意味着你知道一个好的输出是什么样的,一个坏的输出又是什么样的,还要知道一个更好的输入是什么样的。
当你知道某些在编写代码或者执行任务方面做的更好,那实际上确实有助于你对这个模型进行微调,以取得很好的效果。
2.6 首次微调
如果这是你第一次进行微调,建议采取以下步骤:
- 通过对大模型进行提示工程来识别一个任务,可以是像ChatGPT这样的模型。
- 你就像往常一样与chatGPT交互,你会发现,大模型在某些任务上表现还可以,不是很好但是也不是很糟
- 你知道这个任务在可能的范围内是可以实现的,但它不是最好的。而且你希望它在你的任务上表现的更好,所以选择那个任务。只选择一个。
- 为那个任务收集一些输入和输出数据,输入一些文本然后得到一些文本输出。准备一些你输入的文本和对应的输出文本,拿到这样的<输入,输出>样本对。我喜欢使用的样本量可以是1000个,这是一个不错的起始数据量,但要确保这些输入和输出要比之前的大模型的结果好。
- 然后用这些数据微调大模型,感受性能的提升。
2.7 实验 Lab2:Where finetuning fits in
让我们进入试验,你可以探索用于预训练和微调的数据集,这样你就能完全理解这些 <输入,输出>对是什么样的。
例程如下。
首先,导入几个不同的库。第一个库是来自 huggingface的datasets库,函数load_dataset可以从hub中提取数据集并能够运行。
我们提取“The Pile"预训练数据集。"split"指示为训练集而不是测试集。设置streaming=True是因为数据集太大无法一次性下载,所以每次只传输一部分数据。
你可以看到这数据集中有很多看起来像是抓取的数据。这是从互联网上抓取的不同数据集的大杂烩。我想要将其与你将在不同实验中使用的微调数据集进行对比。
接下来我们回去一个有关”问题-答案“对的公司数据集。我们只需读取该JSON文件,查看其中有什么。这是更加结构化的数据,包括大量问题-答案对。而且非常具体,涉及这家公司。
使用这个数据集最简单的方法,是将这些问题和答案连接起来,形成字典,提供给模型。当然,你可以用任何方式准备你的数据。我只是指出一些不同的常见数据格式化和结构化方法,例如问题-答案对,指令-响应对,输入-输出对。你还可以用 “text"将这些文本对连接起来,这非常简单,但有时候就已经足够了。
有时候这还不够,模型还需要提供更多的结构来获得帮助,实际上这与提示工程非常相似。我们再深入一些,你可以使用指令来处理你的数据。例如,构造一个问答提示模板。注意在问题类型token之前有3个”#"号,这种符号让模型知道接下来是什么。模型在看到question之后会期待看到一个问题。它还可以帮助你在对模型进行微调之后处理模型的输出。
我们看看提示模板的效果,查看它与连接的问题-回答的区别。通常将输入和输出保持分开,可以方便评估数据集以及分割数据集。
我们运行一个 for 循环,将所有这些应用到整个数据集中。
存储这些数据最常见的方式通常是 JSON文件。你还可以将这些数据文件上传,以便今后使用。
"""Finetuning data: compare to pretraining and basic preparation"""
import jsonlines
import itertools
import pandas as pd
from pprint import pprint
import datasets
from datasets import load_dataset
"""Look at pretraining data set"""
# Sorry, "The Pile" dataset is currently relocating to a new home and so we can't show you the same example that is in the video. Here is another dataset, the "Common Crawl" dataset.
#pretrained_dataset = load_dataset("EleutherAI/pile", split="train", streaming=True)
pretrained_dataset = load_dataset("c4", "en", split="train", streaming=True)
n = 5
print("Pretrained dataset:")
top_n = itertools.islice(pretrained_dataset, n)
for i in top_n:
print(i)
"""Contrast with company finetuning dataset you will be using"""
filename = "lamini_docs.jsonl"
instruction_dataset_df = pd.read_json(filename, lines=True)
print(instruction_dataset_df)
"""Various ways of formatting your data"""
examples = instruction_dataset_df.to_dict()
text = examples["question"][0] + examples["answer"][0]
print(text)
if "question" in examples and "answer" in examples:
text = examples["question"][0] + examples["answer"][0]
elif "instruction" in examples and "response" in examples:
text = examples["instruction"][0] + examples["response"][0]
elif "input" in examples and "output" in examples:
text = examples["input"][0] + examples["output"][0]
else:
text = examples["text"][0]
prompt_template_qa = """
### Question:
{question}
### Answer:
{answer}"""
question = examples["question"][0]
answer = examples["answer"][0]
text_with_prompt_template = prompt_template_qa.format(question=question, answer=answer)
print(text_with_prompt_template)
prompt_template_q = """
### Question:
{question}
### Answer:"""
num_examples = len(examples["question"])
finetuning_dataset_text_only = []
finetuning_dataset_question_answer = []
for i in range(num_examples):
question = examples["question"][i]
answer = examples["answer"][i]
text_with_prompt_template_qa = prompt_template_qa.format(question=question, answer=answer)
finetuning_dataset_text_only.append({"text": text_with_prompt_template_qa})
text_with_prompt_template_q = prompt_template_q.format(question=question)
finetuning_dataset_question_answer.append({"question": text_with_prompt_template_q, "answer": answer})
pprint(finetuning_dataset_text_only[0])
pprint(finetuning_dataset_question_answer[0])
"""Common ways of storing your data"""
with jsonlines.open(f'lamini_docs_processed.jsonl', 'w') as writer:
writer.write_all(finetuning_dataset_question_answer)
finetuning_dataset_name = "lamini/lamini_docs"
finetuning_dataset = load_dataset(finetuning_dataset_name)
print(finetuning_dataset)
3. 指令微调(Instruction finetuning)
本节我们介绍指令微调。
指令微调是微调的一种,使GPT3能够转变成chatGPT,具有聊天能力。有各种任务可以做,例如推理路由,Copilot辅助编码,与不同的代理人聊天。
3.1 什么是指令微调
指令微调是微调的一种类型,也被称为指令调整(instruction tune)或者指令跟随(instruction following)
LMS只是指示模型遵循指令,并表现的更像一个聊天机器人。这为用户提供了更好的界面与大模型进行交互。例如,利用指令微调将GPT3转变为ChatGPT。这大大提高了AI的使用范围,从几个研究人员到数百万的开发者。
3.2 指令微调数据集
用于指令微调的数据集。你可以利用现有的易得数据,无论是在线的还是专属于你的公司的。包括常见问题解答(FAQs),客户支持对话,Slack消息。这实际上是使用对话数据集,或者指令-回应数据集。
3.3 基于LLM生成指令微调数据
如果你没有指令微调数据集,可以生成数据。
你可以通过使用提示模板,将你的数据转换为更具问答形式或者指令跟随形式的内容。例如,你可以将Readme转化为一个问答对。
你也可以使用另一个LLM来帮你完成。有一种来自斯坦福大学的技术叫Alpaca,它使用ChatGPT来实现这个。
你也可以在不同的开源模型上使用流水线(pipeline)来完成这个任务。
3.4 指令微调泛化能力
关于指令微调最重要的问题,就是它将新的行为教给了大模型。
虽然你可能对"法国的首都是什么"有详细的数据进行微调,因为这些是你可以获取的简单的问题-答案对。
你还可以将这种“问答对”的思想推广到,大模型已经在其现有的预训练阶段中学习过,但是没有提供给大模型进行微调数据集的数据。这实际上是来自chatGPT论文的发现:该模型选择可以回答关于代码的问题,尽管他们在指令微调过程中没有关于这个问题的“问答对”,因为聘请程序员标记数据集实在太贵了。
3.5 微调的步骤
-
微调的步骤,概况起来就是:数据准备、训练和评估,然后不断迭代,重新准备数据来进行改进。
-
改进大模型是一个迭代的过程,并且特别适用于指令微调和其它不同类型的微调。
3.6 Lab3:Instruction tuning lab
现在让我们开始实验,看看指令微调的 Alpaca 数据集,并再次对比进行指令微调和没有进行指令微调的模型。我们还能看到各种不同尺寸的模型。
首先,导入几个库。最重要的是从datasets库中加载数据集的 load_dataset函数。
加载指令微调数据集。这是指定的Alpaca 数据集。我们进行实时的流式传输,因为这个微调数据集很大。
看几个例子,与 Pile 不同,这里的数据具有一定的结构,但只是问答对的关系。Alpaca 的作者提供了两个快捷模板,他们希望该模型能以两种不同类型的提示,与两种不同类型的任务相适应。
chatGPT相比llama2模型来说非常庞大。你也可以尝试一些较小的模型。
这个例程示例了一个指令跟随模型的工作原理,下一步我们讨论如何准备数据,使其可用于模型训练。
"""Instruction-tuning"""
import itertools
import jsonlines
from datasets import load_dataset
from pprint import pprint
from llama import BasicModelRunner
from transformers import AutoTokenizer, AutoModelForCausalLM
from transformers import AutoModelForSeq2SeqLM, AutoTokenizer
"""Load instruction tuned dataset"""
instruction_tuned_dataset = load_dataset("tatsu-lab/alpaca", split="train", streaming=True)
m = 5
print("Instruction-tuned dataset:")
top_m = list(itertools.islice(instruction_tuned_dataset, m))
for j in top_m:
print(j)
"""Two prompt templates"""
prompt_template_with_input = """
Below is an instruction that describes a task, paired with an input that provides further context. Write a response that appropriately completes the request.
### Instruction:
{instruction}
### Input:
{input}
### Response:
"""
prompt_template_without_input = """
Below is an instruction that describes a task. Write a response that appropriately completes the request.
### Instruction:
{instruction}
### Response:
"""
"""Hydrate prompts (add data to prompts)"""
processed_data = []
for j in top_m:
if not j["input"]:
processed_prompt = prompt_template_without_input.format(instruction=j["instruction"])
else:
processed_prompt = prompt_template_with_input.format(instruction=j["instruction"], input=j["input"])
processed_data.append({"input": processed_prompt, "output": j["output"]})
pprint(processed_data[0])
"""Save data to jsonl"""
with jsonlines.open(f'alpaca_processed.jsonl', 'w') as writer:
writer.write_all(processed_data)
"""Compare non-instruction-tuned vs. instruction-tuned models"""
dataset_path_hf = "lamini/alpaca"
dataset_hf = load_dataset(dataset_path_hf)
print(dataset_hf)
non_instruct_model = BasicModelRunner("meta-llama/Llama-2-7b-hf")
non_instruct_output = non_instruct_model("Tell me how to train my dog to sit")
print("Not instruction-tuned output (Llama 2 Base):", non_instruct_output)
instruct_model = BasicModelRunner("meta-llama/Llama-2-7b-chat-hf")
instruct_output = instruct_model("Tell me how to train my dog to sit")
print("Instruction-tuned output (Llama 2): ", instruct_output)
chatgpt = BasicModelRunner("chat-gpt")
instruct_output_chatgpt = chatgpt("Tell me how to train my dog to sit")
print("Instruction-tuned output (ChatGPT): ", instruct_output_chatgpt)
"""Try smaller models"""
tokenizer = AutoTokenizer.from_pretrained("EleutherAI/pythia-70m")
model = AutoModelForCausalLM.from_pretrained("EleutherAI/pythia-70m")
def inference(text, model, tokenizer, max_input_tokens=1000, max_output_tokens=100):
# Tokenize
input_ids = tokenizer.encode(
text,
return_tensors="pt",
truncation=True,
max_length=max_input_tokens)
# Generate
device = model.device
generated_tokens_with_prompt = model.generate(
input_ids=input_ids.to(device),
max_length=max_output_tokens)
# Decode
generated_text_with_prompt = tokenizer.batch_decode(generated_tokens_with_prompt, skip_special_tokens=True)
# Strip the prompt
generated_text_answer = generated_text_with_prompt[0][len(text):]
return generated_text_answer
finetuning_dataset_path = "lamini/lamini_docs"
finetuning_dataset = load_dataset(finetuning_dataset_path)
print(finetuning_dataset)
test_sample = finetuning_dataset["test"][0]
print(test_sample)
print(inference(test_sample["question"], model, tokenizer))
"""Compare to finetuned small model"""
instruction_model = AutoModelForCausalLM.from_pretrained("lamini/lamini_docs_finetuned")
print(inference(test_sample["question"], instruction_model, tokenizer))
"""
Pssst! If you were curious how to upload your own dataset to Huggingface
Here is how we did it
!pip install huggingface_hub
!huggingface-cli login
import pandas as pd
import datasets
from datasets import Dataset
finetuning_dataset = Dataset.from_pandas(pd.DataFrame(data=finetuning_dataset))
finetuning_dataset.push_to_hub(dataset_path_hf)
"""
4. 准备训练数据(Data preparation)
本节我们介绍如何准备数据进行训练。
4.1 准备什么数据
你需要准备什么样的数据呢?有一些好的最佳实践。
-
高质量
首先是高质量的数据,这对于微调来说是最重要的。如果输入垃圾数据进行微调,就会得到垃圾的结果。 -
多样性
其次是多样性。多方面的,多样化的数据是有帮助的。如果所有的输入-输出都是相同的,大模型就开始记忆它们了,然后大模型将开始一遍又一遍的重复说同样的话 -
真实性
很多方法可以创建生成的数据,例如使用LLM生成数据,但实际上拥有真实数据非常重要。
特别针对写作任务,那是因为生成的数据已经具有一定的模式。你可能听说一些检测文本是否AI生成的服务,这就是因为自动生成的数据存在一定的模式,可以被检测出来。
如果训练时使用相同的模式,大模型不一定会学习到新的模式或者新的表达方式。 -
尽可能多的数据
在机器学习中,拥有更多的数据比拥有较少数据更重要。
但是,预训练已经可以解决很多问题,预训练已经从大量数据中学习到了知识,使得大模型有了良好的语言理解能力。因此,对于微调来说,使用尽可能多的数据虽然很重要,但是绝对没有数据质量重要。
4.2 数据准备步骤
我们来看看收集数据的一些步骤:
- 收集指令-响应对,或问题-回答对
- 将这些指令-响应对配对地连接起来,或者添加提示模板
- 对数据进行tokenize,添加填充或者截断,使其大小适合模型输入
- 将这些数据分为训练数据集和测试数据集
4.3 数据向量化
tokenizing的真正含义是什么?
tokenizing 是将文本数据转换为数字的过程,如图所示。转换编码规则不一定是按照字面意思来的,而是基于常见字符的出现频率。
例如,微调,或者说 tokenizing,会将动名词的"ing"映射为编码 278,当你使用相同的tokenizing对其进行解码时,就恢复成为相同的文本“ing”.
有很多不同的 tokenizer。tokenizer与特定的大模型相关联,因为它都是在大模型上进行训练的。如果选择了错误的 tokenizer,模型会非常困惑,因为它期望不同的数字代表不同的字母集和不同的单词,因此要确保使用正确的tokenizer
4.4 Lab4:Data Preparation
我们在实验中学习如何准备数据。例程如下。
首先,还是导入几个库。最重要的是 Huggingface 的 Transformers 库中的 AutoTokenizer类,它会自动找到适合你的模型的正确的 tokenizer。你只要指定模型的名字,就能匹配该模型的正确的 tokenizer。
然后输入一段文本,对该文本进行 tokenize,就得到该文本的编码。tokenizer输出一个包含输入ID的字典,这些ID代表标记。我们再将其解码回文本,于是又得到我们输入的文本内容。这就完成了编码-解码的过程。
在进行tokenize时,经常要进行批量输入。这里给出一些例子,可以直接放入tokenizer中进行批处理。
不同长度的文本,tokenizer 长度也是不同的。但对于模型来说,批次中所有内容长度都必须相同,因为使用固定大小的张量进行操作。
对于长度不足的文本,需要进行填充(padding)。填充式处理可变长度编码文本的策略。你可以指定要表示填充的数字,例如 0,这也是句子结束的标记。当我们选择填充模式时,字符串右侧就会生成很多“0”字符填充,以匹配所需的字符串长度。
模型tokenize也有其所能处理和接受的最大长度。正如在提示工程中,提示长度也有限制。对此,截断(Truncation)是一种处理长文本的策略,使文本和编码变得更短以适合模型的要求。
通常,我们将填充(padding)和截断(Truncation)选项都甚至为True。
下面我们使用准备的文本,运行这个tokenizer。首先将问题与答案连接起来,然后通过tokenizer运行。抓取最大长度,然后截断。
我们将其封装为一个完整的函数,就可以在整个数据集上运行。这就是用于将你的数据集进行tokenizing的功能。
下一步是将数据集分割,运行分割数据集函数,指定测试集的大小为10%。
你可以使用公司的数据集。我们也包含了一些更有趣的数据集,你可以使用它们并定制和训练你的模型。
"""Data preparation"""
import pandas as pd
import datasets
from pprint import pprint
from transformers import AutoTokenizer
"""Tokenizing text"""
tokenizer = AutoTokenizer.from_pretrained("EleutherAI/pythia-70m")
text = "Hi, how are you?"
encoded_text = tokenizer(text)["input_ids"]
print(encoded_text)
decoded_text = tokenizer.decode(encoded_text)
print("Decoded tokens back into text: ", decoded_text)
"""Tokenize multiple texts at once"""
list_texts = ["Hi, how are you?", "I'm good", "Yes"]
encoded_texts = tokenizer(list_texts)
print("Encoded several texts: ", encoded_texts["input_ids"])
"""Padding and truncation"""
tokenizer.pad_token = tokenizer.eos_token
encoded_texts_longest = tokenizer(list_texts, padding=True)
print("Using padding: ", encoded_texts_longest["input_ids"])
encoded_texts_truncation = tokenizer(list_texts, max_length=3, truncation=True)
print("Using truncation: ", encoded_texts_truncation["input_ids"])
tokenizer.truncation_side = "left"
encoded_texts_truncation_left = tokenizer(list_texts, max_length=3, truncation=True)
print("Using left-side truncation: ", encoded_texts_truncation_left["input_ids"])
encoded_texts_both = tokenizer(list_texts, max_length=3, truncation=True, padding=True)
print("Using both padding and truncation: ", encoded_texts_both["input_ids"])
"""Prepare instruction dataset"""
filename = "lamini_docs.jsonl"
instruction_dataset_df = pd.read_json(filename, lines=True)
examples = instruction_dataset_df.to_dict()
if "question" in examples and "answer" in examples:
text = examples["question"][0] + examples["answer"][0]
elif "instruction" in examples and "response" in examples:
text = examples["instruction"][0] + examples["response"][0]
elif "input" in examples and "output" in examples:
text = examples["input"][0] + examples["output"][0]
else:
text = examples["text"][0]
prompt_template = """### Question:
{question}
### Answer:"""
num_examples = len(examples["question"])
finetuning_dataset = []
for i in range(num_examples):
question = examples["question"][i]
answer = examples["answer"][i]
text_with_prompt_template = prompt_template.format(question=question)
finetuning_dataset.append({"question": text_with_prompt_template, "answer": answer})
from pprint import pprint
print("One datapoint in the finetuning dataset:")
pprint(finetuning_dataset[0])
"""Tokenize a single example"""
text = finetuning_dataset[0]["question"] + finetuning_dataset[0]["answer"]
tokenized_inputs = tokenizer(
text,
return_tensors="np",
padding=True
)
print(tokenized_inputs["input_ids"])
max_length = 2048
max_length = min(
tokenized_inputs["input_ids"].shape[1],
max_length)
tokenized_inputs = tokenizer(
text,
return_tensors="np",
truncation=True,
max_length=max_length)
tokenized_inputs["input_ids"]
"""Tokenize the instruction dataset"""
def tokenize_function(examples):
if "question" in examples and "answer" in examples:
text = examples["question"][0] + examples["answer"][0]
elif "input" in examples and "output" in examples:
text = examples["input"][0] + examples["output"][0]
else:
text = examples["text"][0]
tokenizer.pad_token = tokenizer.eos_token
tokenized_inputs = tokenizer(
text,
return_tensors="np",
padding=True,
)
max_length = min(
tokenized_inputs["input_ids"].shape[1],
2048
)
tokenizer.truncation_side = "left"
tokenized_inputs = tokenizer(
text,
return_tensors="np",
truncation=True,
max_length=max_length
)
return tokenized_inputs
finetuning_dataset_loaded = datasets.load_dataset("json", data_files=filename, split="train")
tokenized_dataset = finetuning_dataset_loaded.map(
tokenize_function,
batched=True,
batch_size=1,
drop_last_batch=True
)
print(tokenized_dataset)
tokenized_dataset = tokenized_dataset.add_column("labels", tokenized_dataset["input_ids"])
"""Prepare test/train splits"""
split_dataset = tokenized_dataset.train_test_split(test_size=0.1, shuffle=True, seed=123)
print(split_dataset)
"""Some datasets for you to try"""
finetuning_dataset_path = "lamini/lamini_docs"
finetuning_dataset = datasets.load_dataset(finetuning_dataset_path)
print(finetuning_dataset)
taylor_swift_dataset = "lamini/taylor_swift"
bts_dataset = "lamini/bts"
open_llms = "lamini/open_llms"
dataset_swiftie = datasets.load_dataset(taylor_swift_dataset)
print(dataset_swiftie["train"][1])
"""
This is how to push your own dataset to your Huggingface hub
!pip install huggingface_hub
!huggingface-cli login
split_dataset.push_to_hub(dataset_path_hf)
"""
5. 训练过程(Training process)
本节我们讨论微调的训练过程。训练后可以看到模型在你的任务上的得到改进,成为能够与它聊天的专有模型。
5.1 LLM的训练过程
在LLM中进行训练,训练过程与其它神经网络非常相似。
- 喂入训练数据
- 计算损失函数
- 误差反向传播
- 更新权重
首先喂入训练数据。添加训练数据,计算损失函数。开始时的预测结果是完全错误的。于是通过反向传播模型来更新模型参数,逐渐改善模型的输出结果。
大模型训练有很多超参数。例如,学习速率、学习速率调度器、超参数优化器。
大模型的训练过程(Pytorch)如下,这些只是Pytorch的一般训练过程。
- 首先,要定义迭代次数 epoch。一个epoch是对整个数据集的一次遍历,可能要多次迭代整个数据集。
- 然后,要分批加载数据。将若干数据集合在一起,成为一个batch。
- 输入一个 batch 的数据,前向传播计算模型的损失函数,反向传播计算梯度,更新模型次数
下面我们进一步看看实验中训练过程是如何操作的。
5.2 Lab5:Training
目前很多框架已经封装了很多高级接口,极大简化了大模型训练过程。
有很多优秀的库使训练过程越来越容易,其中一个是Lamini(羊驼) llama library,只需要 3行代码来训练你的模型。
这是托管在外部GPU上的,可以运行任何开源模型。例如我们请求的 41000万参数的模型。你只需要执行 model.train(),然后返回一个仪表盘,并且带有一个模型ID,你可以通过该ID继续调用并进行训练,或者进行推理运行。
本例程的实验,使用 Pythia 7M模型(7000万参数),可以很好的在CPU上运行。所以我们可以看到整个训练过程。
首先,要加载所有这些库。其中一个使带有不同函数的应用程序库 utilities。
我们从训练的不同配置参数开始。有两种导入数据的方式,一个是指定一个特殊的数据集的路径,另一种是指定一个 huggingface路径。把所有选项都放进一个训练配置文件中,传递给模型。
接下来是如前一个例程的 tokenizer。加载 tokenizer,分割数据集。
接下来加载模型,指定模型的名称。
如果你可以使用 GPU,使用这段 Pytorch代码,设置 GPU设备,把模型放到 GPU训练。如果没有GPU,则使用CPU训练。
将这些步骤整合起来,并添加一下推理步骤。
接下来,我们先看一下第一个测试集的微调,将其输入模型。模型回答的方式非常奇怪,并不是真正的答案。然后我们训练模型,进行微调。随着训练的进行,损失函数逐渐减小。训练完成以后,我们把经过微调的模型保存在本地。然后就可以随时加载这个微调模型,不用再从云端下载。我们再看看它在测试集上的表现以及在测试数据点上的表现。我们在这个数据集上对这个我们上传到 HuggingFace 的LaminideDoxFineTune模型进行了两次微调,现在你可以下载并真正使用。
如果你在自己的电脑上尝试,可能需要半个小时到一个小时,取决于你的CPU处理器速度。如果使用 GPU,可能只要几分钟。
我们使用微调的模型,现在的答案要好得多,可以与实际目标答案媲美。但是在最后,还是有重复的内容。你也可以训练更长时间。
有趣的是,你也可以在外部托管的GPU上训练这个模型。提交训练任务,可以通过链接查看工作状态。你还可以注册Lumini,获得你自己的 API密钥,以便能够运行你自己的模型,并更私密地检查所有的训练作业的状态。你还可以与其它人分享你的微调模型。
因此,训练后的微调模型取得了很大进步,接下来我们将继续评估它们。
import datasets
import tempfile
import logging
import random
import config
import os
import yaml
import logging
import time
import torch
import transformers
import pandas as pd
from utilities import *
from transformers import AutoTokenizer
from transformers import AutoModelForCausalLM
from transformers import TrainingArguments
from transformers import AutoModelForCausalLM
from llama import BasicModelRunner
from llama import BasicModelRunner
logger = logging.getLogger(__name__)
global_config = None
# Load the Lamini docs dataset
dataset_name = "lamini_docs.jsonl"
dataset_path = f"/content/{dataset_name}"
use_hf = False
dataset_path = "lamini/lamini_docs"
use_hf = True
# Set up the model, training config, and tokenizer
model_name = "EleutherAI/pythia-70m"
training_config = {
"model": {
"pretrained_name": model_name,
"max_length" : 2048
},
"datasets": {
"use_hf": use_hf,
"path": dataset_path
},
"verbose": True
}
tokenizer = AutoTokenizer.from_pretrained(model_name)
tokenizer.pad_token = tokenizer.eos_token
train_dataset, test_dataset = tokenize_and_split_data(training_config, tokenizer)
print(train_dataset)
print(test_dataset)
# Load the base model
base_model = AutoModelForCausalLM.from_pretrained(model_name)
device_count = torch.cuda.device_count()
if device_count > 0:
logger.debug("Select GPU device")
device = torch.device("cuda")
else:
logger.debug("Select CPU device")
device = torch.device("cpu")
base_model.to(device)
# Define function to carry out inference
def inference(text, model, tokenizer, max_input_tokens=1000, max_output_tokens=100):
# Tokenize
input_ids = tokenizer.encode(
text,
return_tensors="pt",
truncation=True,
max_length=max_input_tokens
)
# Generate
device = model.device
generated_tokens_with_prompt = model.generate(
input_ids=input_ids.to(device),
max_length=max_output_tokens
)
# Decode
generated_text_with_prompt = tokenizer.batch_decode(generated_tokens_with_prompt, skip_special_tokens=True)
# Strip the prompt
generated_text_answer = generated_text_with_prompt[0][len(text):]
return generated_text_answer
# Try the base model
test_text = test_dataset[0]['question']
print("Question input (test):", test_text)
print(f"Correct answer from Lamini docs: {test_dataset[0]['answer']}")
print("Model's answer: ")
print(inference(test_text, base_model, tokenizer))
# Setup training
max_steps = 3
trained_model_name = f"lamini_docs_{max_steps}_steps"
output_dir = trained_model_name
training_args = TrainingArguments(
# Learning rate
learning_rate=1.0e-5,
# Number of training epochs
num_train_epochs=1,
# Max steps to train for (each step is a batch of data)
# Overrides num_train_epochs, if not -1
max_steps=max_steps,
# Batch size for training
per_device_train_batch_size=1,
# Directory to save model checkpoints
output_dir=output_dir,
# Other arguments
overwrite_output_dir=False, # Overwrite the content of the output directory
disable_tqdm=False, # Disable progress bars
eval_steps=120, # Number of update steps between two evaluations
save_steps=120, # After # steps model is saved
warmup_steps=1, # Number of warmup steps for learning rate scheduler
per_device_eval_batch_size=1, # Batch size for evaluation
evaluation_strategy="steps",
logging_strategy="steps",
logging_steps=1,
optim="adafactor",
gradient_accumulation_steps = 4,
gradient_checkpointing=False,
# Parameters for early stopping
load_best_model_at_end=True,
save_total_limit=1,
metric_for_best_model="eval_loss",
greater_is_better=False
)
model_flops = (
base_model.floating_point_ops(
{
"input_ids": torch.zeros(
(1, training_config["model"]["max_length"])
)
}
)
* training_args.gradient_accumulation_steps
)
print(base_model)
print("Memory footprint", base_model.get_memory_footprint() / 1e9, "GB")
print("Flops", model_flops / 1e9, "GFLOPs")
trainer = Trainer(
model=base_model,
model_flops=model_flops,
total_steps=max_steps,
args=training_args,
train_dataset=train_dataset,
eval_dataset=test_dataset,
)
# Train a few steps
training_output = trainer.train()
# Save model locally
save_dir = f'{output_dir}/final'
trainer.save_model(save_dir)
print("Saved model to:", save_dir)
finetuned_slightly_model = AutoModelForCausalLM.from_pretrained(save_dir, local_files_only=True)
finetuned_slightly_model.to(device)
# Run slightly trained model
test_question = test_dataset[0]['question']
print("Question input (test):", test_question)
print("Finetuned slightly model's answer: ")
print(inference(test_question, finetuned_slightly_model, tokenizer))
test_answer = test_dataset[0]['answer']
print("Target answer output (test):", test_answer)
# Run same model trained for two epochs
finetuned_longer_model = AutoModelForCausalLM.from_pretrained("lamini/lamini_docs_finetuned")
tokenizer = AutoTokenizer.from_pretrained("lamini/lamini_docs_finetuned")
finetuned_longer_model.to(device)
print("Finetuned longer model's answer: ")
print(inference(test_question, finetuned_longer_model, tokenizer))
# Run much larger trained model and explore moderation
bigger_finetuned_model = BasicModelRunner(model_name_to_id["bigger_model_name"])
bigger_finetuned_output = bigger_finetuned_model(test_question)
print("Bigger (2.8B) finetuned model (test): ", bigger_finetuned_output)
count = 0
for i in range(len(train_dataset)):
if "keep the discussion relevant to Lamini" in train_dataset[i]["answer"]:
print(i, train_dataset[i]["question"], train_dataset[i]["answer"])
count += 1
print(count)
# Explore moderation using small model
base_tokenizer = AutoTokenizer.from_pretrained("EleutherAI/pythia-70m")
base_model = AutoModelForCausalLM.from_pretrained("EleutherAI/pythia-70m")
print(inference("What do you think of Mars?", base_model, base_tokenizer))
# Now try moderation with finetuned small model
print(inference("What do you think of Mars?", finetuned_longer_model, tokenizer))
# Finetune a model in 3 lines of code using Lamini
model = BasicModelRunner("EleutherAI/pythia-410m")
model.load_data_from_jsonlines("lamini_docs.jsonl")
model.train(is_public=True)
out = model.evaluate()
lofd = []
for e in out['eval_results']:
q = f"{e['input']}"
at = f"{e['outputs'][0]['output']}"
ab = f"{e['outputs'][1]['output']}"
di = {'question': q, 'trained model': at, 'Base Model' : ab}
lofd.append(di)
df = pd.DataFrame.from_dict(lofd)
style_df = df.style.set_properties(**{'text-align': 'left'})
style_df = style_df.set_properties(**{"vertical-align": "text-top"})
style_df
6. 评估与迭代(Evaluation and iteration)
下一步是评估,看看微调模型的表现如何。
这个步骤很重要。人工智能的关键在于迭代,这有助于让你随着时间的推移改善你的模型。
6.1 评估难点
评估生成模型是非常困难的,很难用明确的指标来衡量模型的性能。模型不断进步,原有的指标很难跟上。结果就是,人类评估通常是最可靠的方法之一,这就需要特定领域的专家来评估大模型的输出结果。
一个好的的测试数据集也是非常重要的,首先要保证测试数据集是高质量的、准确的、泛化的,确保覆盖大模型的不同测试案例
另一种越来越流行的测试方式是 Elo对比测试,相当于多个模型之间的A/B测试。Elo排名是用于国际象棋的。通过这种比较可以了解哪些模型表现良好或者不好。
6.2 基准测试
一个常见的开放 LLM 基准是一套不同的评估方法,这种方法将许多不同的可能的评估方法综合在一起,以平均值的方式对大模型进行综合排名,常见的基准测试包括:
- ARC是一套小学问题
- HellaSwag是一个常识测试
- MMLU涵盖了许多小学科目
- TruthfulQA衡量大模型再现网上常见谎言的能力
这些是研究人员开发的一组基准,现在已经在这个常见的评估套件中使用。
6.3 误差分析
另一个用于分析和评估大模型的方法就是误差分析,它是对错误进行分类,并跟踪常见的错误、灾难性错误
由于误差分析通常需要先训练大模型,或者已经有一个预训练的基础模型,通过误差分析可以知道问题在哪里,这样你就知道使用什么样的数据进行微调可以提升大模型的性能
误差分析常见的问题和处理方法包括:
- 拼写错误:修正数据集,确保拼写正确
- 太长:确保数据集比较简洁,确保大模型输出不会太啰嗦
- 重复:截断处理,通过使用停止标记来修复。另外,尽量使数据集具有多样性,不太重复。
6.4 Lab6:Evalution
现在进行一个实验,在一个测试数据集上运行该模型。在LLM基准测试上运行。
例程如下。
实际上这可以只用一行代码来完成,以批处理的方式在整个测试数据集上运行你的模型,非常高效。
加载模型,实例化,然后让它在你的整个测试数据集上运行。在GPU上自动进行批处理,速度非常快。
# Technically, there are very few steps to run it on GPUs, elsewhere (ie. on Lamini).
finetuned_model = BasicModelRunner(
"lamini/lamini_docs_finetuned"
)
finetuned_output = finetuned_model(
test_dataset_list # batched!
)
下面使使用 CPU 运行的详细例程。
首先加载测试数据集。打印问题答案对,看看一组数据的内容。
然后加载模型,从 Huggingface 中获取微调模型。
加载基本的评估指标,根据这两个字符串是否完全匹配来判断。当然这不是一个有效的用于阅读任务的评估指标,在分类任务中更有意义。这里只是示例,也可以运行不同的评估指标进行测试。
注意模型评估时,要将 dropout 这样的参数禁用。
然后运行推理函数来生成输出。
运行10次,结果时精确匹配的数量为0。由于这是生成任务,这样的结果并不意外。
如果你对ARC标准感兴趣,可以尝试使用 ARC 基准测试。
在运行评估例程时,其实不必太过关注这些基准测试的性能,它们不一定与你的使用需求相关。而你实际关心的,是对于你的使用案例来说,微调模型的什么方面。正如你所看到的,微调模型能够基本上适应大量不同的任务。需要用许多不同的方式来评估它们。
import datasets
import tempfile
import logging
import random
import config
import os
import yaml
import logging
import difflib
import pandas as pd
import transformers
import datasets
import torch
from tqdm import tqdm
from utilities import *
from transformers import AutoTokenizer, AutoModelForCausalLM
logger = logging.getLogger(__name__)
global_config = None
dataset = datasets.load_dataset("lamini/lamini_docs")
test_dataset = dataset["test"]
print(test_dataset[0]["question"])
print(test_dataset[0]["answer"])
model_name = "lamini/lamini_docs_finetuned"
tokenizer = AutoTokenizer.from_pretrained(model_name)
model = AutoModelForCausalLM.from_pretrained(model_name)
# Setup a really basic evaluation function
def is_exact_match(a, b):
return a.strip() == b.strip()
model.eval()
def inference(text, model, tokenizer, max_input_tokens=1000, max_output_tokens=100):
# Tokenize
tokenizer.pad_token = tokenizer.eos_token
input_ids = tokenizer.encode(
text,
return_tensors="pt",
truncation=True,
max_length=max_input_tokens
)
# Generate
device = model.device
generated_tokens_with_prompt = model.generate(
input_ids=input_ids.to(device),
max_length=max_output_tokens
)
# Decode
generated_text_with_prompt = tokenizer.batch_decode(generated_tokens_with_prompt, skip_special_tokens=True)
# Strip the prompt
generated_text_answer = generated_text_with_prompt[0][len(text):]
return generated_text_answer
# Run model and compare to expected answer
test_question = test_dataset[0]["question"]
generated_answer = inference(test_question, model, tokenizer)
print(test_question)
print(generated_answer)
answer = test_dataset[0]["answer"]
print(answer)
exact_match = is_exact_match(generated_answer, answer)
print(exact_match)
# Run over entire dataset
n = 10
metrics = {'exact_matches': []}
predictions = []
for i, item in tqdm(enumerate(test_dataset)):
print("i Evaluating: " + str(item))
question = item['question']
answer = item['answer']
try:
predicted_answer = inference(question, model, tokenizer)
except:
continue
predictions.append([predicted_answer, answer])
#fixed: exact_match = is_exact_match(generated_answer, answer)
exact_match = is_exact_match(predicted_answer, answer)
metrics['exact_matches'].append(exact_match)
if i > n and n != -1:
break
print('Number of exact matches: ', sum(metrics['exact_matches']))
df = pd.DataFrame(predictions, columns=["predicted_answer", "target_answer"])
print(df)
# Evaluate all the data
evaluation_dataset_path = "lamini/lamini_docs_evaluation"
evaluation_dataset = datasets.load_dataset(evaluation_dataset_path)
pd.DataFrame(evaluation_dataset)
# Try the ARC benchmark
# This can take several minutes
!python lm-evaluation-harness/main.py --model hf-causal --model_args pretrained=lamini/lamini_docs_finetuned --tasks arc_easy --device cpu
import os
os.listdir("lm-evaluation-harness")
7. 总结与实践(Consideration on getting started now)
这是我们的最后一节。我们介绍你在开始时需要考虑的一些事项,一些实用的提示,和一些更高级的训练方法。
7.1 微调的一些实用方法
- 想清楚任务是什么
- 收集与任务相关的输入/输出数据,并将数据结构化
- 如果没有足够多的数据,可以利用提示模板生成一些数据
- 可以先对小型模型(推荐4亿-10亿参数)进行微调,了解模型的表现
- 改变提供给模型的数据量,以了解数据量对模型性能的影响有多大
- 评估模型性能
- 收集更多的数据来改进模型
- 增加任务的复杂度,或增加模型的大小,以提高在更复杂任务上的性能
7.2 微调和模型大小
-
任务的复杂度
要求大模型输出的 token 越多,复杂度会越高
”阅读“类的文本提取任务相对于“写作”类的文本提取任务更简单
聊天,写电子邮件,写代码,这些任务更复杂,因为模型生成了更多的 token。总体而言是更难的任务。 -
越困难的任务往往需要更大的模型来处理
-
一种处理更困难的任务的方式,是将多个任务组合在一起,要求大模型同时完成多个任务,而不仅仅是一个任务。
这可能意味着让大模型同时做几件事情,或者有多个步骤。 -
大模型运行所需要的硬件资源大致如下所示
在CPU上运行大模型并不理想,建议使用较高性能的模型。建议的第一行是一台 1v100的GPU,16GB内存,这在AWS上是可用的,也适用于其它云平台,可以运行一个拥有70亿参数的模型进行推理。但训练需要更多的内存来存储梯度和优化器,因此实际上该配置可以适应一个十亿参数的模型。
如果希望适配更大参数的模型,可以参见其它可用的选项。
7.3 参数高效微调
如果这对你还不够,你想要用更大的模型,可以使用参数高效微调(PEFT)。这是一种不同的方法,可以在使用参数和训练模型方面更加高效。
LoRA,代表低级别适应性。
LoRA 的作用是大幅度减少你需要训练的参数数量,即你需要训练的权重数量,降低了10000倍,这导致GPU需要的内存减少了3倍。尽管在微调过程中获得的准确度稍微低一些,这仍然是一种更高效的方式,并且在最后获得相同的推理延迟。
LoRA究竟发生了什么?
微调实际上是在模型的某些层中训练新的权重。你正在冻结主要的预训练权重,这些权重显示为蓝色,只微调这些橙色的权重——LoRA权重。
新的权重是原始权重变化的分级分解矩阵,依据背后的数学原理,你可以分别训练它们,更新对应的预训练权重。但在推理时,可以将它们合并回原有的主预训练权重。这样可以更高效地获得经过微调的模型。
LoRA 让我感到兴奋的是将其适用于新任务。这就意味着你可以使用一位客户的数据来训练一个LoRA模型,然后再用另一位客户的数据训练另一个模型,然后能将它们每个模型合并,并在需要推理时使用它们。
通过这个教程,你了解了什么是微调,它适合哪些任务,以及为什么它很重要。
现在,微调是你的一种工具。你以及完成了从数据准备,训练,到评估模型的所有步骤,
我非常非常激动,我希望看到你能用它来创造什么。
原文链接:
https://learn.deeplearning.ai/finetuning-large-language-models/lesson/1/introduction
版权声明:
『ChatGPT Prompt Engineering for Developers』是DeepLearning.AI出品的免费课程,版权属于DeepLearning.AI(https://www.deeplearning.ai/)。
本文是对该课程内容的翻译整理,只作为教育用途,不作为任何商业用途。
Translated and organized by youcans@qq.com, 2024
Crated:2023-06-15
翻译整理:西安邮电大学 黄杉 (https://github.com/youcans)
更多推荐
所有评论(0)